Strategic Insights for Developing an AI Roadmap That Delivers Value
Developing an AI roadmap can be daunting for businesses. How do you ensure your AI initiatives deliver real value? This guide provides strategic insights for creating an effective AI roadmap. We’ll cover key components of a successful roadmap, steps for development, and strategies for execution. You’ll learn how to align AI initiatives with business goals, overcome common challenges, and measure success. By following these insights, you’ll be equipped to develop an AI roadmap that drives tangible results for your organization.
Essential Key Takeaways for Developing an Effective AI Roadmap
- AI roadmaps align business goals with AI initiatives to deliver value across sectors
- Effective AI implementation requires strategic planning, resource allocation, and stakeholder engagement
- Organizations must address data quality, change management, and risk mitigation challenges in AI adoption
- Continuous monitoring, adaptation, and performance analysis are crucial for AI roadmap success
- Embracing advanced technologies and anticipating regulatory changes shape future AI roadmap development strategies
Understanding the Purpose of an AI Roadmap
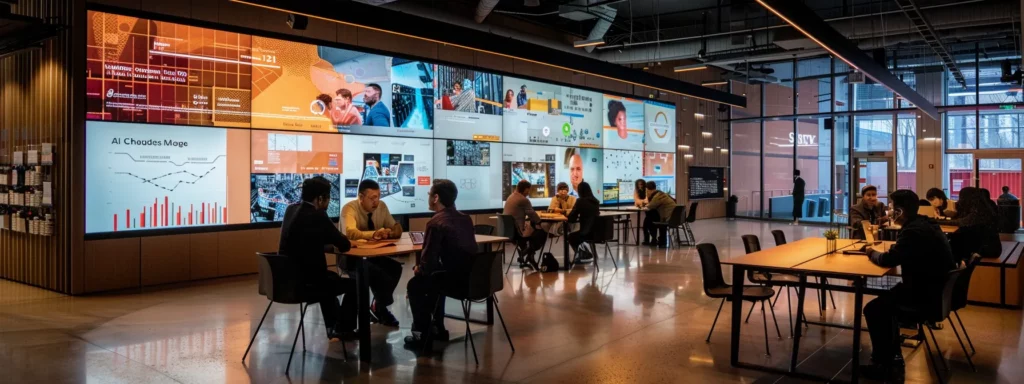
An AI roadmap serves as a strategic guide for organizations implementing artificial intelligence technologies. It focuses on identifying business goals, aligning AI initiatives with organizational strategy, and recognizing key stakeholders. This approach ensures that AI implementation, including machine learning and natural language processing, delivers value across various sectors such as health care and law. A well-crafted AI roadmap provides a clear path for integrating AI solutions that align with an organization’s
and long-term objectives.
Identifying Business Goals to Guide AI Implementation
Identifying business goals is a crucial first step in developing an effective AI roadmap. Organizations must clearly define their objectives, whether they involve improving prediction accuracy, enhancing customer experiences, or streamlining operations. A fractional chief ai officer can help businesses align these goals with AI capabilities, ensuring the roadmap addresses specific organizational needs.
An AI roadmap should outline how artificial intelligence will support and advance the organism’s overall strategy. By connecting AI initiatives to tangible business outcomes, companies can prioritize projects, allocate resources efficiently, and measure the impact of their AI investments. This approach enables businesses to create a roadmap that delivers real value and drives sustainable growth through AI adoption.
Aligning AI Initiatives With Organizational Strategy
Aligning AI initiatives with organizational strategy requires a careful assessment of existing infrastructure and skills. Organizations must evaluate their current capabilities in areas such as PyTorch development and data management to identify gaps and opportunities for AI integration. This alignment ensures that AI projects contribute directly to the organization’s goals, whether in improving patient care through explainable artificial intelligence or enhancing
.
Successful alignment also involves developing a skilled workforce capable of implementing and maintaining AI systems. Organizations should invest in training programs to build expertise in key areas like PyTorch and machine learning, while also considering the ethical implications of AI deployment. By fostering a culture of continuous learning and innovation, companies can ensure their AI initiatives remain in sync with evolving organizational strategies and patient needs:
Alignment Factor | Key Considerations |
---|---|
Infrastructure | Assess PyTorch compatibility, data storage capabilities |
Skills | Identify gaps in AI expertise, plan training programs |
Ethics | Ensure explainable AI for patient trust and compliance |
Strategy | Link AI projects to organizational goals and KPIs |
Recognizing Key Stakeholders in the AI Journey
Recognizing key stakeholders is crucial for successful AI strategy implementation, as it ensures ethical considerations and diverse perspectives are incorporated into the AI roadmap. Stakeholders from various departments, including data science and data analysis teams, contribute valuable insights on how AI can address specific challenges such as disease prediction and treatment optimization. By involving these key players early in the process, organizations can develop a more comprehensive and effective AI strategy.
Effective stakeholder engagement also facilitates the integration of deep learning technologies across different organizational levels. This collaborative approach helps identify potential roadblocks and opportunities for AI adoption, ensuring that the roadmap aligns with both technical capabilities and business objectives. By fostering open communication among stakeholders, organizations can create an AI roadmap that balances innovation with responsible implementation, ultimately delivering greater value to the business and its customers.
Critical Components of an Effective AI Roadmap
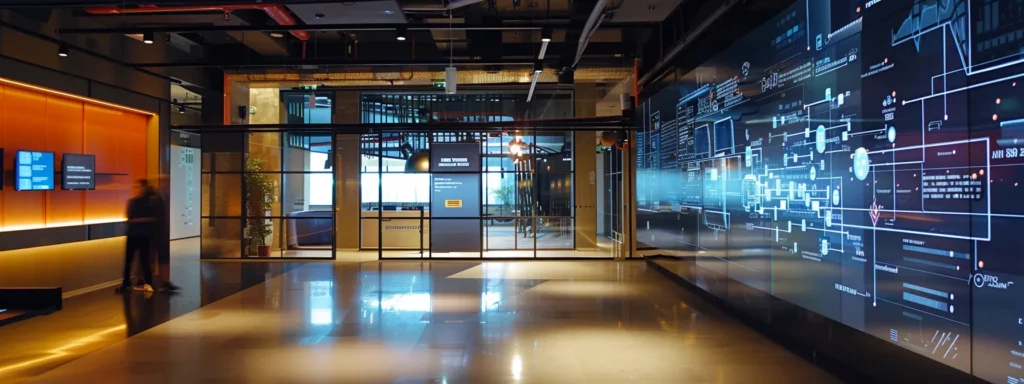
Effective AI roadmaps require strategic planning and resource allocation. Key components include defining objectives and success metrics, assessing current data and technological capabilities, and outlining required resources and budgets. These elements form the foundation for successful AI implementation, incorporating advanced techniques in algebra, computer vision, and intelligent sampling to drive business value and innovation.
Defining AI Objectives and Success Metrics
Defining AI objectives and success metrics forms a critical foundation for an effective AI roadmap. Organizations must establish clear, measurable goals that align with their overall business strategy and AI policy. These objectives should focus on leveraging AI to extract value from unstructured data, enhance knowledge management, and improve decision-making processes.
Success metrics for AI initiatives should be specific, quantifiable, and tied to business outcomes. Organizations can track key performance indicators such as improved customer attention, increased newsletter engagement, or enhanced data processing efficiency. By setting concrete benchmarks, companies can evaluate the impact of their AI implementations and make data-driven adjustments to their roadmap as needed.
Assessing Current Data and Technological Capabilities
Assessing current data and technological capabilities is crucial for developing an effective AI roadmap. Organizations must evaluate their existing infrastructure, including hardware and software systems, to determine their readiness for AI implementation. This assessment involves analyzing the organization’s proficiency in key areas such as linear algebra, computer science, and statistics, which form the foundation for advanced AI techniques.
A comprehensive evaluation of data quality, quantity, and accessibility is essential for successful AI initiatives. Organizations should conduct thorough research to understand their data landscape, identifying potential gaps and opportunities for improvement. This process enables businesses to make informed decisions about necessary investments in data collection, storage, and processing capabilities, ensuring a solid foundation for
and innovation.
Outlining Required Resources and Budgeting for AI Projects
Outlining required resources and budgeting for AI projects is crucial for successful implementation. Organizations must assess their intellectual property needs, including software licenses and data ownership, to mitigate risks and foster innovation. This process involves evaluating the probability of success for different AI initiatives and allocating resources accordingly, ensuring a balanced approach to risk management and technological advancement.
Effective budgeting for AI projects requires consideration of potential biases in data and algorithms, as well as investments in talent acquisition and training. Organizations should allocate funds for ongoing monitoring and adjustment of AI systems to maintain accuracy and fairness. By prioritizing resources for ethical AI development, companies can reduce the risk of reputational damage and legal challenges while maximizing the value of their AI investments.
Steps for Creating a Comprehensive AI Roadmap
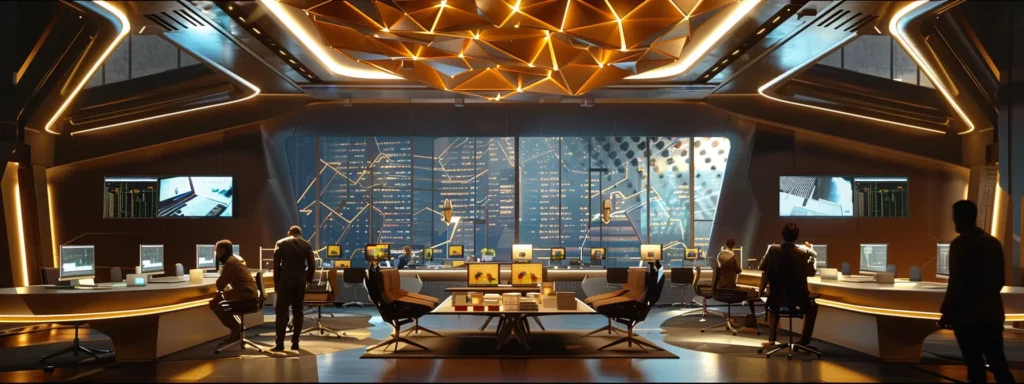
Creating a comprehensive AI roadmap involves critical steps: conducting a gap analysis for AI readiness, prioritizing use cases based on impact and feasibility, and developing a timeline for deliverables. This process requires a strong foundation in data governance, mathematics, and big data management. Organizations must leverage the expertise of data scientists to navigate these steps effectively, ensuring their AI initiatives align with business objectives and governance frameworks.
Conducting a Gap Analysis for AI Readiness
Conducting a gap analysis for AI readiness involves assessing an organization’s current capabilities against its desired AI implementation goals. This process identifies areas where improvements in machine learning algorithms, statistical models, and data infrastructure are needed. Organizations evaluate their existing resources, including hardware, software, and human expertise, to determine the steps required for
.
A comprehensive gap analysis examines various aspects of AI readiness, including data quality, processing power, and staff competencies. Organizations often use specialized tools and frameworks to measure their maturity in key areas such as data governance, algorithm development, and ethical AI practices. The results of this analysis guide the development of targeted strategies to bridge identified gaps and enhance overall AI capabilities:
- Assess current AI capabilities and resources
- Identify gaps in technology, skills, and processes
- Develop strategies to address identified gaps
- Prioritize improvements based on business objectives
- Create action plans for enhancing AI readiness
Prioritizing AI Use Cases Based on Impact and Feasibility
Prioritizing AI use cases requires organizations to evaluate potential projects based on their impact and feasibility. This process involves assessing each use case’s potential return on investment, alignment with business objectives, and technical complexity. By systematically ranking use cases, companies can focus their resources on initiatives that offer the greatest value while considering their current AI capabilities.
To effectively prioritize AI use cases, organizations often employ scoring frameworks that consider factors such as data availability, technical requirements, and potential business impact. This approach enables decision-makers to balance ambitious AI goals with practical considerations, ensuring a realistic and achievable roadmap. Regular reassessment of priorities allows companies to adapt their AI strategy as technologies evolve and new opportunities emerge.
Developing a Timeline for AI Deliverables
Developing a timeline for AI deliverables is a crucial step in creating a comprehensive
. Organizations must establish realistic timelines that account for the complexity of AI projects, resource availability, and potential challenges. This process involves breaking down larger initiatives into manageable milestones and setting clear deadlines for each phase of implementation.
Effective timeline development requires input from various stakeholders, including data scientists, IT professionals, and business leaders. Organizations should consider factors such as data preparation, model training, testing, and deployment when estimating project durations. Regular progress reviews and timeline adjustments ensure the AI roadmap remains flexible and aligned with evolving business needs:
Timeline Phase | Key Activities | Estimated Duration |
---|---|---|
Data Preparation | Collection, cleaning, and structuring | 2-4 weeks |
Model Development | Algorithm selection, training, and validation | 4-8 weeks |
Testing and Refinement | Performance evaluation and optimization | 2-4 weeks |
Deployment | Integration and user training | 2-4 weeks |
Strategies for Executing Your AI Roadmap
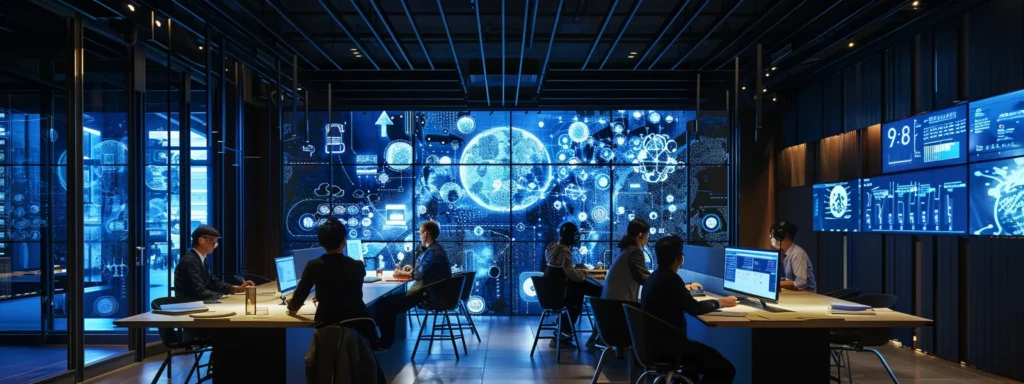
Executing an AI roadmap requires strategic approaches to ensure successful implementation. Organizations should foster a culture of innovation and collaboration, implement agile methodologies for AI development, and establish continuous monitoring and
. These strategies enable businesses to navigate the complexities of AI integration, maximize resource utilization, and maintain alignment with evolving technological landscapes and business objectives.
Fostering a Culture of Innovation and Collaboration
Fostering a culture of innovation and collaboration is essential for successful AI roadmap execution. Organizations must create an environment that encourages cross-functional teamwork, idea sharing, and experimentation. This approach enables businesses to leverage diverse expertise, accelerate problem-solving, and drive creative AI solutions that align with strategic objectives.
To promote innovation and collaboration, companies should implement structured programs for knowledge sharing and skill development. These initiatives may include hackathons, innovation labs, and mentorship programs that bring together data scientists, business analysts, and domain experts. By fostering a collaborative ecosystem, organizations can overcome silos, enhance
across departments, and cultivate a workforce ready to embrace AI-driven transformation:
- Establish cross-functional AI teams
- Organize regular innovation workshops
- Implement a knowledge sharing platform
- Encourage experimentation and calculated risk-taking
- Recognize and reward innovative AI solutions
Implementing Agile Methodologies for AI Development
Implementing agile methodologies for AI development enables organizations to adapt quickly to changing requirements and deliver value incrementally. This approach involves breaking down AI projects into smaller, manageable sprints, allowing for frequent reassessment and adjustment of priorities. By adopting agile practices, companies can reduce risks associated with AI implementation and maintain flexibility in their roadmap execution.
Agile methodologies in AI development foster collaboration between data scientists, developers, and business stakeholders. Regular sprint reviews and retrospectives provide opportunities for continuous improvement and ensure that AI solutions remain aligned with business objectives. This iterative approach allows organizations to validate assumptions, gather feedback, and refine AI models more efficiently:
Agile Practice | Benefits for AI Development |
---|---|
Sprint Planning | Prioritize AI features and set clear goals |
Daily Stand-ups | Enhance team communication and problem-solving |
Sprint Reviews | Demonstrate AI progress and gather feedback |
Retrospectives | Identify areas for process improvement |
Ensuring Continuous Monitoring and Adaptation of the Roadmap
Ensuring continuous monitoring and adaptation of the AI roadmap is crucial for long-term success. Organizations must establish robust feedback mechanisms to track the performance of AI initiatives against predefined metrics and business objectives. This ongoing evaluation allows companies to identify areas for improvement, adjust resource allocation, and refine their
in response to changing market conditions and technological advancements.Regular reviews of the AI roadmap enable organizations to maintain alignment with evolving business priorities and capitalize on new opportunities. Companies should implement a systematic approach to gathering insights from AI projects, including data on model accuracy, user adoption, and business impact. By fostering a culture of continuous learning and adaptation, organizations can optimize their AI investments and drive sustained
through their roadmap execution.
Evaluating the Success of Your AI Initiatives
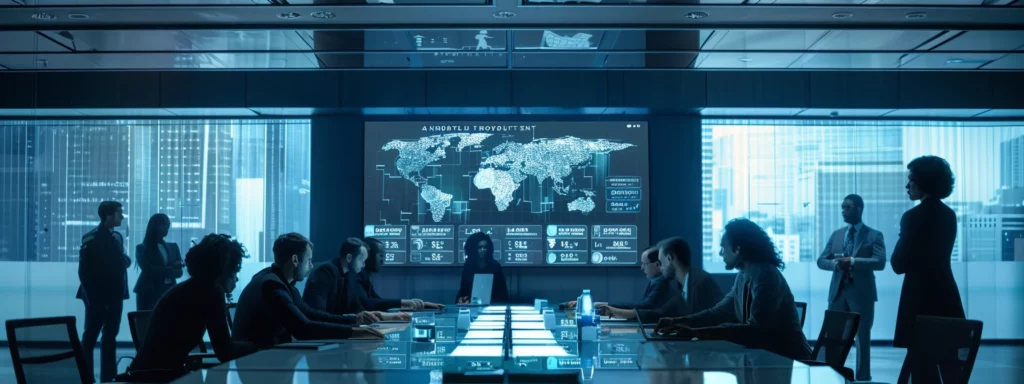
Evaluating AI initiative success is crucial for refining and optimizing an organization’s
. This process involves analyzing performance against defined metrics, iterating on the roadmap based on feedback, and sharing results with stakeholders. By systematically assessing AI projects, companies can identify areas for improvement, adjust strategies, and ensure ongoing alignment with business objectives.
Analyzing Performance Against Defined Metrics
Analyzing performance against defined metrics is crucial for evaluating AI initiative success. Organizations must establish clear, quantifiable indicators that align with their strategic objectives and measure the impact of AI implementations. These metrics may include productivity improvements, cost reductions, or customer satisfaction scores, providing tangible evidence of AI’s value to the business.
Regular performance assessments enable organizations to identify areas for improvement and optimize their AI strategies. By comparing actual results against predefined benchmarks, companies can make data-driven decisions about resource allocation and future AI investments. This analytical approach ensures that AI initiatives remain aligned with business goals and deliver measurable value over time.
Iterating on the AI Roadmap Based on Feedback
Iterating on the AI roadmap based on feedback is essential for maintaining its relevance and effectiveness. Organizations should establish regular review cycles to assess the performance of AI initiatives and gather insights from stakeholders. This feedback loop enables companies to identify areas for improvement, adjust priorities, and refine their AI strategies to better align with evolving business needs and technological advancements.
Effective iteration involves analyzing both quantitative and qualitative feedback to drive continuous improvement. Organizations should leverage data analytics tools to track key performance indicators and user engagement metrics. By combining this data with stakeholder input, companies can make informed decisions about resource allocation, project prioritization, and potential pivots in their AI roadmap. This iterative approach ensures that AI initiatives remain agile and responsive to changing market conditions:
Iteration Step | Key Activities | Expected Outcome |
---|---|---|
Feedback Collection | Surveys, interviews, data analysis | Comprehensive insights |
Performance Assessment | KPI evaluation, benchmarking | Identified improvement areas |
Strategy Refinement | Reprioritization, resource reallocation | Optimized AI roadmap |
Implementation | Updating projects, communicating changes | Enhanced AI initiatives |
Sharing Results and Lessons Learned With Stakeholders
Sharing results and lessons learned with stakeholders is crucial for maintaining transparency and driving continuous improvement in AI initiatives. Organizations should prepare comprehensive reports that highlight key achievements, challenges faced, and insights gained from AI implementations. These reports should present data-driven evidence of AI’s impact on business objectives, fostering stakeholder
and support for future AI investments.Regular stakeholder communication enables organizations to align AI strategies with evolving business needs and expectations. Companies should organize periodic briefings or workshops to discuss AI project outcomes, addressing both successes and areas for improvement. This open dialogue promotes a culture of learning and collaboration, empowering stakeholders to contribute valuable insights and shape the
within the organization.
Addressing Common Challenges in AI Roadmap Development
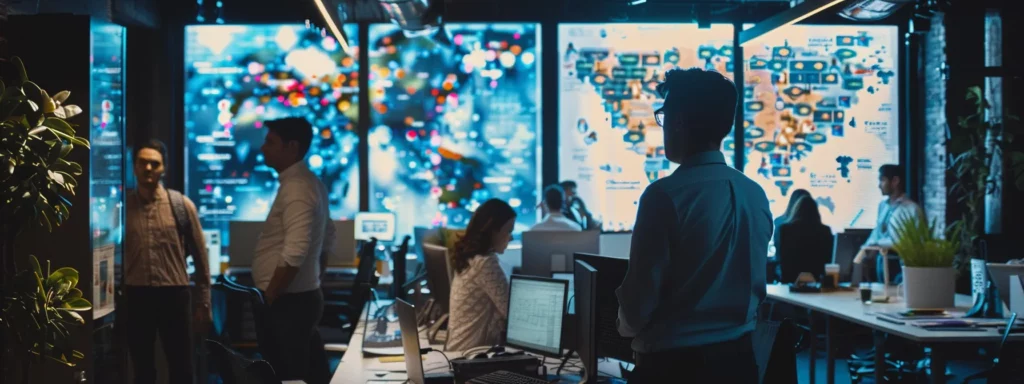
Developing an effective AI roadmap often faces challenges in data quality, change management, and risk mitigation. Organizations must address data availability issues, navigate cultural shifts during AI adoption, and implement strategies to minimize risks associated with AI implementation. By tackling these challenges head-on, businesses can create robust AI roadmaps that deliver tangible value and drive
.
Overcoming Data Quality and Availability Issues
Organizations must address data quality and availability issues to develop effective AI roadmaps. This involves implementing robust data governance frameworks, establishing data cleansing processes, and investing in data integration technologies. By prioritizing data quality, companies can ensure their AI models are built on reliable foundations, leading to more accurate predictions and insights.
To overcome data availability challenges, organizations should explore alternative data sources and leverage data partnerships. This may include utilizing external datasets, implementing data-sharing agreements with partners, or developing synthetic data generation techniques. By expanding their data ecosystem, companies can enhance the scope and effectiveness of their AI initiatives:
Challenge | Solution | Impact |
---|---|---|
Data Quality | Implement data governance | Improved model accuracy |
Data Availability | Explore alternative sources | Enhanced AI capabilities |
Data Integration | Invest in integration tools | Streamlined AI development |
Navigating Change Management in AI Adoption
Navigating change management in AI adoption requires organizations to develop comprehensive strategies that address both technical and cultural aspects of transformation. Companies must create clear communication plans to articulate the benefits of AI implementation, addressing concerns and managing expectations across all levels of the organization. This approach helps build trust and buy-in among employees, fostering a culture that embraces AI-driven
.
Successful change management in AI adoption also involves providing adequate training and support to employees as they adapt to new AI-enabled processes and tools. Organizations should invest in upskilling programs that enhance AI literacy and data competencies across the workforce. By empowering employees with the knowledge and skills to leverage AI effectively, companies can accelerate adoption rates and maximize the value of their AI investments.
Mitigating Risks Associated With AI Implementation
Mitigating risks associated with AI implementation requires organizations to develop comprehensive risk management strategies. Companies must conduct thorough assessments to identify potential ethical, legal, and operational risks related to AI deployment. This process involves evaluating data privacy concerns, algorithmic bias, and regulatory compliance issues to ensure responsible AI adoption.
Organizations should establish robust governance frameworks and monitoring systems to address AI-related risks effectively. These mechanisms enable continuous oversight of AI models, allowing for timely detection and mitigation of issues such as model drift or unexpected outcomes. By implementing rigorous testing protocols and maintaining human oversight, companies can enhance the reliability and trustworthiness of their AI systems, minimizing potential negative impacts on business operations and stakeholder trust.
Future Trends in AI and Their Impact on Roadmap Development
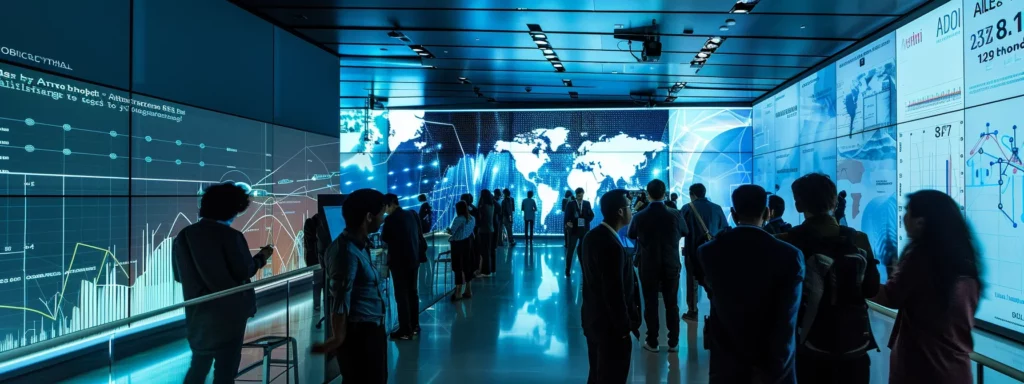
Future AI trends significantly influence roadmap development strategies. Organizations must embrace advanced technologies and methodologies, anticipate regulatory changes and ethical considerations, and prepare for evolving AI applications. These factors shape how businesses plan, implement, and optimize their AI initiatives, ensuring long-term value and competitive advantage in an increasingly AI-driven landscape.
Embracing Advanced AI Technologies and Methodologies
Organizations developing AI roadmaps must embrace advanced technologies and methodologies to stay competitive. Cutting-edge approaches like federated learning and edge AI enable businesses to process data more efficiently while maintaining privacy and reducing latency. These technologies allow companies to extend AI capabilities to resource-constrained devices, opening new possibilities for
and personalized user experiences.
Integrating quantum computing and neuromorphic hardware into AI roadmaps can lead to significant performance improvements. These technologies have the potential to solve complex optimization problems and process vast amounts of data at unprecedented speeds. By incorporating these advanced AI technologies into their roadmaps, organizations can position themselves at the forefront of innovation and unlock new opportunities for growth and efficiency.
Anticipating Regulatory Changes and Ethical Considerations
Organizations developing AI roadmaps must anticipate regulatory changes and ethical considerations to ensure long-term sustainability. As AI technologies advance, governments worldwide are implementing stricter regulations to protect
and prevent algorithmic bias. Companies should proactively integrate compliance measures into their AI strategies, addressing issues such as data protection, transparency, and fairness in AI decision-making processes.
Ethical considerations play a crucial role in shaping AI roadmaps, as businesses face increasing scrutiny over the societal impact of their AI systems. Organizations should establish ethical AI frameworks that prioritize human-centered design, accountability, and responsible innovation. By incorporating ethical guidelines into their AI development processes, companies can build trust with stakeholders, mitigate reputational risks, and create AI solutions that align with societal values and expectations.
Preparing for the Evolving Landscape of AI Applications
Organizations must prepare for the evolving landscape of AI applications by incorporating flexibility and scalability into their roadmaps. As AI technologies advance, new use cases emerge across industries, presenting opportunities for innovation and competitive advantage. Companies should design their AI strategies to accommodate rapid integration of emerging applications, such as autonomous systems, advanced natural language processing, and predictive analytics.
To stay ahead in the evolving AI landscape, organizations should foster a culture of continuous learning and experimentation. This approach enables businesses to quickly adapt to new AI applications and leverage them for value creation. By establishing
dedicated to exploring and evaluating emerging AI technologies, companies can identify potential applications that align with their strategic objectives and integrate them seamlessly into their existing AI roadmaps.
Resources for Enhancing Your AI Roadmap
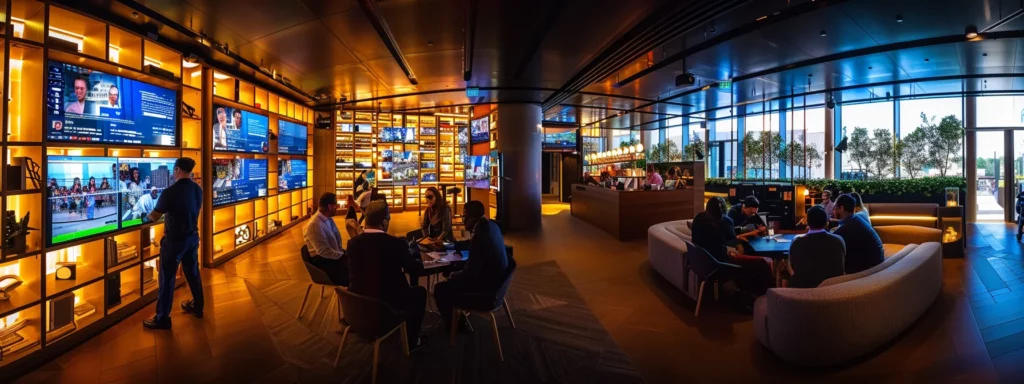
Enhancing AI roadmaps requires strategic use of resources. This section explores tools and technologies for AI development, key literature informing best practices, and networking opportunities within the AI community. These resources provide valuable insights and support for organizations looking to optimize their AI strategies and stay ahead in the rapidly evolving field of artificial intelligence.
Recommended Tools and Technologies for AI Development
Organizations developing AI roadmaps can leverage a range of powerful tools and technologies to enhance their development processes. Popular frameworks such as TensorFlow and PyTorch provide robust foundations for building and deploying machine learning models, while cloud-based platforms like AWS SageMaker and Google Cloud AI offer scalable infrastructure for AI development. These tools enable businesses to streamline their
, from data preparation to model training and deployment.
For organizations focusing on natural language processing, libraries such as spaCy and NLTK offer comprehensive solutions for text analysis and understanding. Additionally, automated machine learning platforms like H2O.ai and DataRobot can accelerate the model development process, allowing businesses to rapidly prototype and iterate on AI solutions. By selecting the appropriate tools and technologies, companies can significantly improve the efficiency and effectiveness of their AI roadmap implementation.
Key Literature and Research to Inform Best Practices
Organizations developing AI roadmaps can benefit from key literature and research that inform best practices. Publications such as “Artificial Intelligence: A Modern Approach” by Stuart Russell and Peter Norvig provide comprehensive overviews of AI concepts and methodologies.
from reputable sources like Gartner and McKinsey offer valuable insights into emerging trends and practical applications of AI across various sectors.
Academic journals such as “Nature Machine Intelligence” and “Journal of Artificial Intelligence Research” present cutting-edge findings that can shape AI strategies. These resources, combined with case studies from successful AI implementations, offer a solid foundation for organizations to develop effective AI roadmaps. By staying informed about the latest research and industry benchmarks, companies can make data-driven decisions and align their AI initiatives with proven best practices:
Resource Type | Examples | Benefits |
---|---|---|
Textbooks | “Artificial Intelligence: A Modern Approach” | Comprehensive AI foundations |
Industry Reports | Gartner, McKinsey | Emerging trends and applications |
Academic Journals | “Nature Machine Intelligence” | Cutting-edge research findings |
Case Studies | Successful AI implementations | Practical insights and lessons learned |
Networking Opportunities Within the AI Community
Networking opportunities within the AI community offer valuable resources for organizations developing AI roadmaps. Industry conferences such as NeurIPS, ICML, and AI Summit provide platforms for professionals to exchange ideas, learn about cutting-edge technologies, and connect with potential partners. These events often feature workshops, panel discussions, and presentations that can inform AI strategies and best practices.
Online communities and forums, such as Kaggle and AI Stack Exchange, enable knowledge sharing and collaboration among AI practitioners. Participating in these platforms allows organizations to tap into collective expertise, solve complex problems, and stay updated on emerging trends. Additionally, local AI meetups and hackathons provide opportunities for hands-on learning and networking with peers in the field: AI literacy
Networking Opportunity | Benefits | Examples |
---|---|---|
Industry Conferences | Knowledge exchange, trend insights | NeurIPS, ICML, AI Summit |
Online Communities | Collaborative problem-solving | Kaggle, AI Stack Exchange |
Local Meetups | Hands-on learning, peer networking | AI hackathons, tech meetups |
Frequently Asked Questions
How does an AI roadmap differ from a traditional technology roadmap?
An AI roadmap focuses on integrating artificial intelligence capabilities into business processes, emphasizing data strategy, algorithm development, and ethical considerations. Unlike traditional technology roadmaps, it prioritizes machine learning, automation, and cognitive technologies to drive innovation and competitive advantage in a rapidly evolving AI landscape.
What are the key steps in creating an effective AI roadmap?
Creating an effective AI roadmap involves assessing business needs, identifying AI opportunities, prioritizing initiatives, establishing data infrastructure, securing leadership buy-in, developing talent, planning implementation phases, and setting measurable goals. Regular evaluation and adaptation are crucial for long-term success.
How can businesses measure the success of their AI initiatives?
Businesses can measure AI success through key performance indicators (KPIs) aligned with their goals. These may include improved efficiency, cost savings, revenue growth, customer satisfaction, or decision-making accuracy. Regular assessments, data analysis, and feedback loops help track progress and refine AI strategies over time.
What are common challenges faced when developing an AI roadmap?
Developing an AI roadmap often faces challenges like unclear business objectives, data quality issues, skill gaps, resistance to change, integration complexities, and ethical concerns. Organizations struggle to align AI initiatives with overall strategy, secure resources, and manage expectations while navigating the
.
How do emerging AI trends impact roadmap development for businesses?
Emerging AI trends significantly influence business roadmap development by reshaping strategies, priorities, and resource allocation. Companies must adapt their plans to incorporate AI-driven innovations, address potential disruptions, and leverage new opportunities for growth, efficiency, and competitive advantage in an increasingly AI-driven marketplace.
Conclusion
Developing a strategic AI roadmap is crucial for organizations seeking to harness the power of artificial intelligence and drive tangible business value. By aligning AI initiatives with organizational goals, prioritizing high-impact use cases, and addressing key challenges such as data quality and change management, companies can create a clear path for successful
. Continuous evaluation and adaptation of the roadmap, coupled with a focus on ethical considerations and emerging technologies, ensure long-term sustainability and competitive advantage in an increasingly AI-driven landscape. Leveraging the right tools, staying informed about industry best practices, and actively engaging with the AI community are essential steps for organizations to optimize their AI strategies and unlock the full potential of this transformative technology.