Measuring ROI of AI Adoption: Essential Metrics for Business Impact
Measuring the return on investment (ROI) of AI adoption is crucial for businesses, yet many struggle to quantify its impact. This article explores essential metrics for evaluating AI effectiveness and business value. We’ll cover techniques for calculating AI ROI, discuss key performance indicators, and examine challenges in measurement. By the end, you’ll have practical insights to assess your AI initiatives’ success and make
about future investments.
Set Clear Goals for Measuring AI ROI
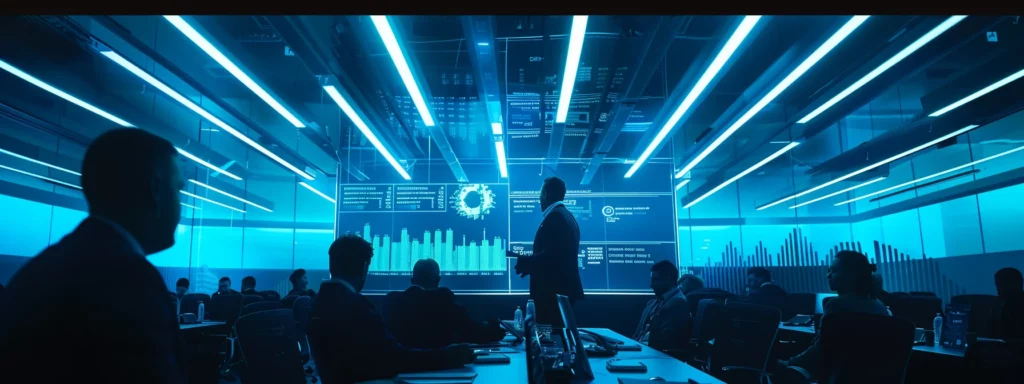
Setting clear goals for measuring AI ROI is crucial for successful implementation. This process involves identifying specific business objectives, aligning AI initiatives with overall strategy, and defining success metrics. By focusing on these areas, organizations can effectively evaluate the impact of AI on their software engineering, data validation, and informationtechnology processes, ultimately improving patient care and data management.
Identify Specific Business Objectives for AI Implementation
Identifying specific business objectives for AI implementation requires a comprehensive stakeholderanalysis. Organizations must engage key decision-makers to pinpoint areas where AI can drive business transformation and enhance data security. This process ensures that AI initiatives align with the company‘s overall
and address critical pain points.Once stakeholders have defined clear objectives, organizations can develop a precise formula for measuring AI’s impact. This formula should incorporate quantifiable metrics related to operational efficiency, cost reduction, and revenue generation. By establishing these metrics upfront, businesses can accurately track the AI ROI.
and adjust their strategies accordingly.
Effective goal-setting for AI implementation also involves prioritizing data security and privacy concerns. Organizations must balance their desire for innovation with the need to protect sensitive information, including passwords and personal data. By incorporating security considerations into their AI objectives, businesses can mitigate risks and build trust with customers and partners. AI strategy
Align AI Initiatives With Overall Business Strategy
Aligning AI initiatives with overall business strategy is crucial for maximizing ROI and driving sustainable growth. Organizations must carefully assess how AI can support key business objectives, such as improving wealthmanagement services or accelerating drug discovery processes. This alignment ensures that AI investments contribute directly to the company‘s long-term goals and financial performance.
Effective alignment requires collaboration between AI experts and business leaders to identify high-impact areas for implementation. For example, in the financial sector, AI can enhance risk assessment models and optimize investment strategies, potentially increasing dollar returns for clients. By focusing on these strategic applications, companies can justify AI investments and demonstrate tangible benefits to stakeholders.
Organizations must also consider regulatory compliance when aligning AI initiatives with business strategy. As AI technologies evolve, companies need to stay informed about changing regulations and adapt their implementations accordingly. This proactive approach helps mitigate risks and ensures that AI-driven innovations in areas like drug discovery or wealthmanagement remain compliant with industry standards. regulatory compliancestrategic alignment with ai and smart kpis
Define Success Metrics Before AI Adoption
Defining success metrics before AI adoption is crucial for transparency and measuring the impact on business operations. Organizations should establish clear, quantifiable indicators that align with their strategic goals. These metrics may include improved efficiency in telecommunications, enhanced code quality through tools like GitHub Copilot, or increased productivity in specific departments. strategic alignment with AI and smart KPIs.
Leadership teams must collaborate to identify key performance indicators (KPIs) that reflect the desired outcomes of AI implementation. These KPIs should be specific, measurable, and directly tied to business objectives. For example, a company might track the reduction in customer service response times or the increase in successful software deployments following AI adoption.
Regular evaluation of these predefined metrics enables organizations to assess the effectiveness of their AI initiatives and make data-driven decisions. By consistently monitoring and analyzing these indicators, businesses can identify areas for improvement, optimize their AI strategies, and demonstrate tangible value to stakeholders. This approach fosters a culture of continuous improvement and ensures that AI investments deliver measurable returns.
Understanding AI ROI Calculation Techniques
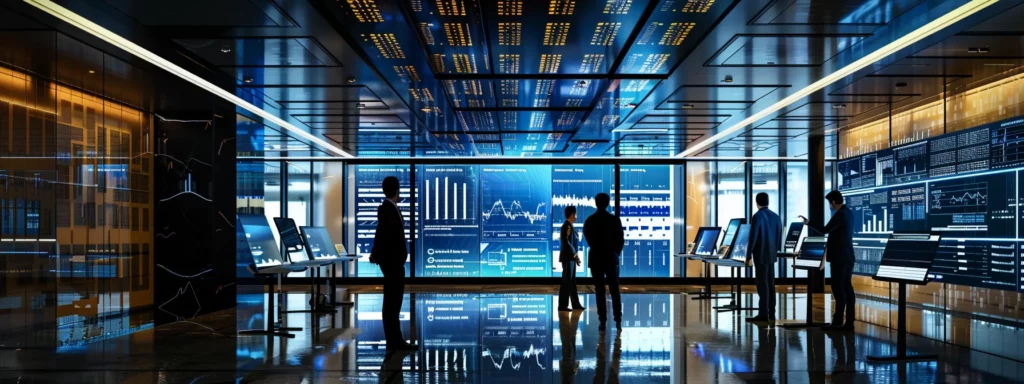
Understanding AI ROI calculation techniques is crucial for businesses evaluating AI investments. This section explores common approaches to calculate AI ROI, utilizing cost-benefit analysis, and integrating time savings and productivity gains. By focusing on robotic process automation, valuemanagement, and data analysis, organizations can gain insights into the intelligence and efficiency improvements offered by AI adoption. artificial intelligence roi
Explore Common Approaches to Calculate AI ROI
Organizations employ various methodologies to calculate AI ROI, focusing on both financial and non-financial metrics. These approaches often involve comprehensive data collection and analysis to assess the impact of AI implementation on equity, operational efficiency, and overall business performance. By utilizing robust calculation techniques, companies can accurately measure the success of their AI initiatives and identify areas for improvement. AI ROI
One common approach to AI ROI calculation involves evaluating the direct financial impact of AI adoption. This methodology examines factors such as cost savings, revenue growth, and return on equity to quantify the monetary benefits of AI implementation. Finance teams play a crucial role in this process, working closely with data scientists and business analysts to develop accurate models for assessing AI ROI.
.
Another essential aspect of AI ROI calculation involves considering the potential risks and costs associated with AI failure. Organizations must factor in the expenses related to systemdowntime, data breaches, or algorithmic errors when evaluating the overall return on investment. By incorporating these risk factors into their ROI calculations, companies can make more informed decisions about AI adoption and allocate resources more effectively.
Utilize Cost-Benefit Analysis for AI Investments
Cost-benefit analysis serves as a crucial tool for evaluating AI investments, enabling organizations to assess the potential impact on operational efficiency and business growth. By comparing the anticipated costs of AI implementation with projected benefits, companies can make informed decisions about resource allocation and prioritize initiatives that offer the highest return on investment.
Effective cost-benefit analysis for AI investments often involves simulation techniques to model various scenarios and predict outcomes. These simulations help organizations estimate the potential impact of AI adoption on key performance indicators, such as resourceconsumption and productivity gains, providing valuable insights for decision-makers.
Organizations must also consider intangible benefits when conducting cost-benefit analyses for AI investments, such as improved brandloyalty and customer satisfaction. While these factors may be challenging to quantify, they play a significant role in determining the long-term success of AI initiatives and should be factored into the overall evaluation process.
Integrate Time Savings and Productivity Gains in ROI Calculation
Integrating time savings and productivity gains into AI ROI calculations provides a comprehensive view of the technology‘s impact on workforceefficiency. Organizations can quantify these benefits by measuring the reduction in task completion times and the increase in output per employee. The World Economic Forum estimates that AI could create up to 97 million new jobs by 2025, highlighting its potential to boost overall productivity and
.
To accurately assess time savings, companies should conduct before-and-after analyses of processes impacted by AI implementation. This approach helps identify specific areas where AI acts as a valuable asset, streamlining operations and freeing up human resources for higher-value tasks. By quantifying these improvements, organizations can calculate the present value of long-term productivity gains resulting from AI adoption. AI strategy
Productivity gains from AI often extend beyond immediate time savings, impacting overall business performance. Companies should consider factors such as improved decision-making capabilities, reduced error rates, and enhanced customer satisfaction when evaluating AI’s ROI. These broader improvements contribute significantly to long-term business value and should be factored into comprehensive ROI calculations to provide a more accurate picture of AI’s impact.
Essential Metrics for Evaluating AI Effectiveness
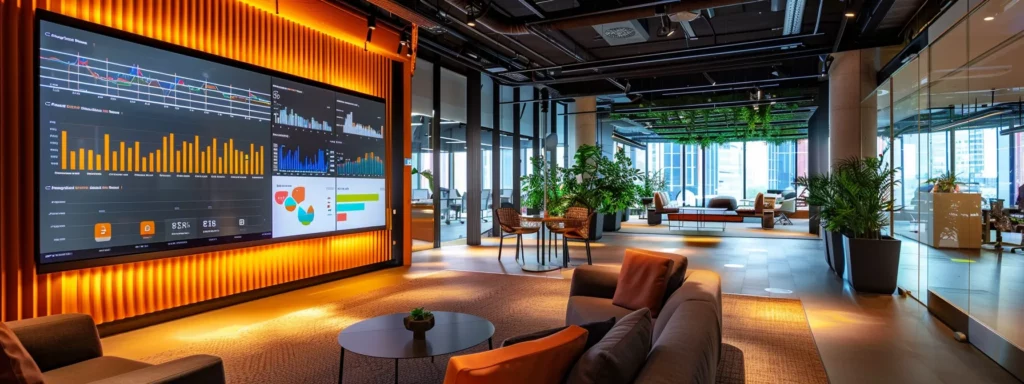
Evaluating AI effectiveness requires comprehensive metrics that align with business objectives. This section explores key performance indicators for measuring efficiency improvements, cost reductions, customer satisfaction, and revenue growth related to AI adoption. By focusing on these metrics, organizations can gain valuable insights into their
efforts, enhancing software development processes and refining marketing strategies through.
.
Measure Efficiency Improvements and Cost Reductions
Measuring efficiency improvements and cost reductions is crucial for evaluating AI effectiveness. Organizations can track metrics such as reduced operational costs, increased throughput, and improved resource allocation to quantify the impact of AI adoption. By implementing predictive maintenance systems, companies can minimize downtime and optimize asset utilization, directly contributing to cost savings and operational efficiency.
AI-driven prediction models enable businesses to forecast demand, optimize inventory levels, and streamline supply chains. These capabilities lead to significant cost reductions and improved efficiency across various industries, including wealthmanagement and manufacturing. By adopting a data-driven mindset, organizations can leverage AI to make more informed decisions and allocate resources more effectively. AI capabilities
Generative artificial intelligence technologies offer new opportunities for efficiency gains and cost reductions. These systems can automate content creation, productdesign, and even software development processes, significantly reducing time-to-market and associated costs. By measuring the impact of generative AI on workflowefficiency and resource utilization, businesses can quantify the ROI of their AI investments and identify areas for further optimization.
Assess Customer Satisfaction and Engagement Metrics
Assessing customer satisfaction and engagement metrics is crucial for evaluating AI effectiveness in improving the overall customerexperience. Organizations can utilize AI-powered sentiment analysis tools to gauge customerfeedback across various touchpoints, providing valuable insights into the impact of AI-driven initiatives on customer satisfaction levels.
AI-enabled chatbots and virtual assistants play a significant role in enhancing customer engagement and satisfaction. By tracking metrics such as response times, query resolution rates, and customerfeedback scores, businesses can measure the effectiveness of these AI solutions in providing seamless and personalized customer support, ultimately contributing to
.
growth.
Integrating AI into business intelligence systems allows companies to analyze customerbehavior patterns and preferences more effectively. By leveraging these insights, organizations can design targeted marketing campaigns and personalized product recommendations, leading to improved customer engagement and loyalty. Additionally, AI-powered cybersecurity measures can enhance customer trust by protecting sensitive data and ensuring a secure online experience.
Track Innovation and Revenue Growth Related to AI
Tracking innovation and revenue growth related to AI adoption requires comprehensive evaluation metrics. Organizations can measure the number of AI-driven product launches, patents filed, and successful mergers and acquisitions to gauge innovation levels. These indicators provide insights into how AI implementation contributes to overall business growth and market
.
Revenue growth attributed to AI can be assessed by analyzing salesdata, customer acquisition rates, and market share increases. Companies should focus on calculating the cost savings and additional revenue generated through AI-enabled processes, such as personalized marketing campaigns or optimized pricing strategies. This approach helps quantify the direct financial impact of AI adoption on the bottom line.
Evaluating synergy between AI initiatives and existing business operations is crucial for understanding long-term value creation. Organizations can track metrics such as cross-departmental collaboration, improved decision-making speed, and enhanced resource allocationefficiency. By assessing these factors, businesses can identify areas where AI contributes to overall organizational effectiveness and drives
.
.
Evaluating AI Business Value Beyond Financial Metrics
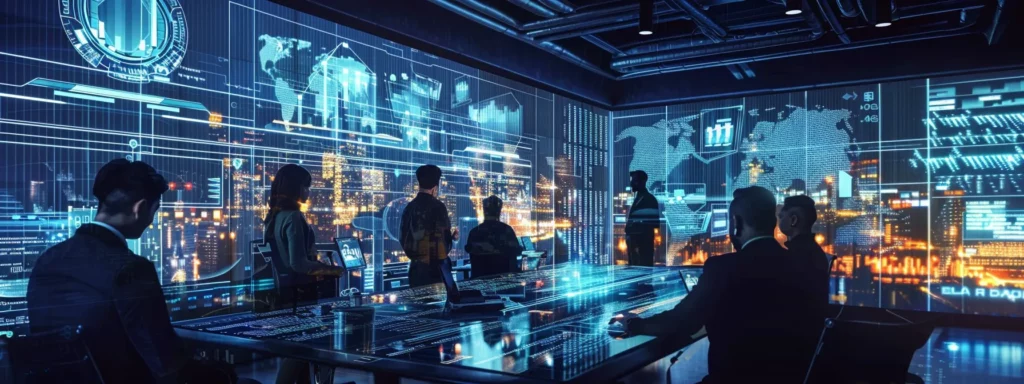
Evaluating AI’s business value extends beyond financial metrics, encompassing employee productivity, market positioning, and long-term strategic benefits. This section explores how AI impacts enterprise business operations, fostering creativity and collaboration while addressing regulatory compliance challenges. By examining these factors, organizations can gain a comprehensive understanding of AI’s role in driving innovation and competitive advantage through cloud computing and other advanced technologies.
Consider Employee Productivity and Satisfaction Levels
In the evolving landscape of AI adoption, organizations must consider employee productivity and satisfaction levels as key indicators of business value. AI technologies can streamline workflows, automate repetitive tasks, and enhance decision-making processes, leading to increased productivity across various departments. By measuring changes in employee output and efficiency, companies can gauge the impact of AI on their workforce‘s
.
.
The future of employment in an AI-driven environment requires a focus on employee satisfaction and engagement. As AI takes over routine tasks, employees can dedicate more time to creative and strategic initiatives, potentially increasing job satisfaction. Organizations should monitor metrics such as employee retention rates, job satisfaction surveys, and career progression to assess the overall impact of AI on their workforce.
Knowledgemanagement and skilldevelopment play crucial roles in maximizing AI’s benefits for employee productivity. Companies can create a continuous learningculture by implementing AI-powered training programs and knowledge-sharing platforms. By tracking employee participation in these initiatives and measuring the resulting improvements in job performance, organizations can quantify the long-term value of AI investments in human capital development.
Analyze Market Positioning and Competitive Advantage Gained
AI adoption significantly enhances market positioning by enabling companies to offer personalized advertising and improved customer service. Organizations leveraging AI-driven insights can tailor their brand messaging and product offerings to meet specific customer needs, resulting in increased market share and customerloyalty.
In the health sector, AI-powered diagnostic tools and predictive analytics give healthcare providers a competitive edge. By reducing diagnostic errors and improving treatment outcomes, these organizations can establish themselves as industry leaders, attracting more patients and partnerships with pharmaceutical companies.
AI implementation also contributes to competitive advantage by minimizing downtime and optimizing operational efficiency. Companies utilizing AI for predictive maintenance and supply chainmanagement can ensure consistent service delivery, outperforming competitors who experience frequent disruptions or inventory shortages.
can play a crucial role in enhancing these AI capabilities.
Examine Long-Term Strategic Benefits of AI Adoption
Examining the long-term strategic benefits of AI adoption reveals significant improvements in systemarchitecture and overall business relevance. Organizations that implement AI-driven solutions can enhance their software-as-a-service offerings, providing more sophisticated and personalized experiences for clients. This strategic approach to AI adoption positions companies for sustained growth and competitiveness in rapidly evolving markets.
AI adoption enables businesses to develop adaptive and scalable architectures that can evolve with changing market demands. By integrating AI into core systems, organizations can create more flexible and responsive infrastructures, facilitating rapid innovation and deployment of new services. This architectural agility allows companies to stay ahead of competitors and maintain relevance in dynamic industries. AI capabilities
The long-term strategic benefits of AI adoption extend to improved decision-making processes and operational efficiency. As AI systems continuously learn and adapt, they provide increasingly accurate insights and predictions, enabling organizations to make data-driven decisions with greater confidence. This enhanced decision-making capability, combined with AI-driven process optimization, leads to sustained improvements in productivity and cost–effectiveness across various business functions.
Challenges in Measuring AI ROI and Effectiveness
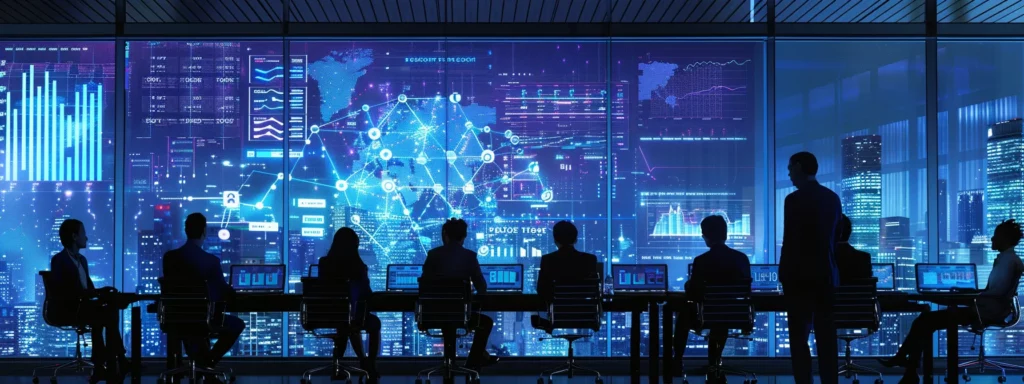
Measuring AI ROI presents significant challenges for businesses. Data quality issues can hinder accurate assessment, while resistance to evaluation practices may impede progress. Uncertainty in predicting long-term impacts, including effects on sales and internal rate of return, further complicates ROI calculations. Organizations must address these obstacles to effectively gauge AI’s business impact and ensure confidence in their
.
Identify Data Quality Issues Hindering Accurate Assessment
Data quality issues pose significant challenges in accurately assessing AI ROI, introducing uncertainty into the evaluation process. Organizations often struggle with incomplete, inconsistent, or outdated datasets, which can lead to skewed results and unreliable performance metrics. This lack of high-quality data hinders companies’ ability to gain a competitive advantage through AI implementation, as the insights derived from flawed data may not accurately reflect the true impact of AI initiatives.
Effective risk assessment and resource allocation in AI projects depend heavily on the availability of clean, reliable data. When data quality is compromised, organizations may misallocate resources or underestimate potential risks associated with AI adoption. This can result in suboptimal decision-making and reduced confidence in AI-driven strategies, ultimately impacting the overall return on investment.
Addressing data quality issues requires a comprehensive approach that goes beyond traditional data management practices. Organizations must implement robust data governance frameworks, invest in data cleansing and enrichment tools, and foster a data-driven culture throughout the company. By prioritizing data quality, businesses can reduce the paper trail of inaccurate assessments and build a solid foundation for measuring
with greater precision and confidence.
Overcome Resistance to AI Measurement and Evaluation Practices
Overcoming resistance to AI measurement and evaluation practices requires a comprehensive approach to project management. Organizations must educate stakeholders about the importance of assessing AI initiatives, emphasizing how accurate measurements contribute to improved decision-making and resource allocation. By demonstrating the link between effective evaluation and successful AI development, companies can build support for robust measurement practices.
Implementing standardized evaluation frameworks can help address resistance to AI measurement. Engineering teams should collaborate with data scientists to develop consistent metrics for assessing neural network performance across different projects. This approach ensures that language models and other AI systems are evaluated uniformly, facilitating meaningful comparisons and fostering a culture of continuous improvement.
Effective communication is crucial in overcoming resistance to AI measurement practices. Project managers should clearly articulate the benefits of evaluation to all team members, highlighting how accurate assessments can lead to more efficient development processes and better outcomes. By framing measurement as a tool for enhancing AI capabilities rather than a burden, organizations can
of.
.
.
Mitigate Uncertainty in Predicting Long-Term AI Impacts
Organizations can mitigate uncertainty in predicting long-term AI impacts by developing robust forecasting models that incorporate key performance indicators across various business functions. By analyzing historical data on recruitment, customerloyalty, and debtmanagement, companies can identify trends and patterns that inform more accurate long-term projections. This approach enables businesses to make data-driven decisions and adjust their
accordingly.
Implementing a culture of continuous learning and adaptation is crucial for managing long-term AI impacts. Companies should establish feedback loops that capture insights from AI implementations and use them to refine future predictions. By fostering a culture that values ongoing evaluation and improvement, organizations can enhance their ability to anticipate and respond to the evolving impacts of AI on their operations and market position.
Collaboration with industry peers and academic institutions can provide valuable insights into long-term AI impacts. By participating in research initiatives and sharing anonymized data, companies can contribute to a broader understanding of AI’s effects on business landscapes. This collaborative approach helps organizations benchmark their AI performance against industry standards and gain a more comprehensive view of potential long-term outcomes.
Case Studies: Successful AI ROI Measurement Practices
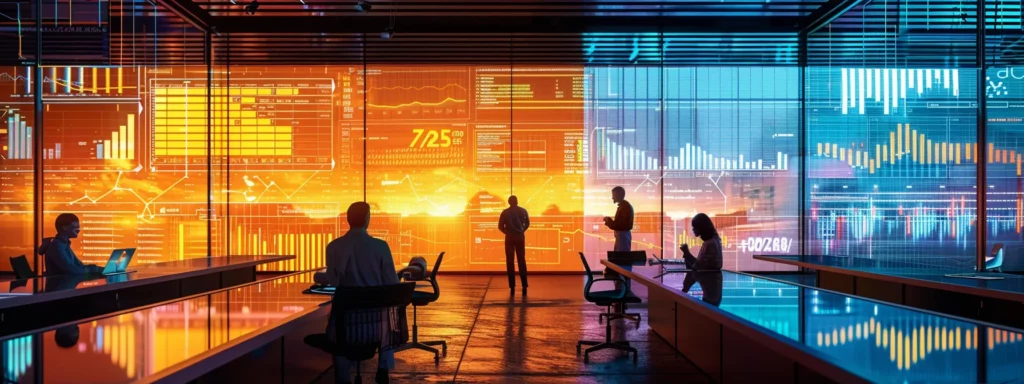
Case studies of successful AI ROI measurement practices offer valuable insights into effective implementation strategies. This section examines businesses demonstrating tangible returns from AI adoption, analyzes strategies employed by industry leaders, and extracts key lessons from success stories. By exploring these examples, organizations can gain practical knowledge to enhance their own AI governance, improve scalability, and develop robust tools for measuring
across various time horizons.
Review Examples of Businesses Demonstrating ROI From AI
PwC‘s implementation of AI-driven budgetforecasting tools demonstrates significant ROI, with reported accuracy improvements of up to 30% in financial projections. By leveraging machinelearning algorithms to analyze historical data and market trends, PwC optimized resource allocation and reduced budget overruns, resulting in substantial cost savings for their clients.
A leading e-commerce company achieved remarkable ROI through AI-powered product recommendation systems built on AWSinfrastructure. The AI-driven app increased customer engagement by 40% and boosted cross-selling opportunities, leading to a 25% rise in average order value. This success highlights the potential of AI in enhancing customer experiences and driving
.
A global manufacturing firm reported substantial ROI from implementing AI-based predictive maintenance systems. By leveraging sensor data and machinelearning algorithms, the company reduced equipment downtime by 35% and maintenance costs by 20%. This proactive approach to assetmanagement improved operational efficiency and provided valuable information for
.
.
Analyze Strategies Employed by Leaders in AI Adoption
Leaders in AI adoption prioritize building comprehensive ecosystems that integrate AI across various business functions. These organizations focus on creating synergies between AI technologies and existing processes, optimizing inventorymanagement and cash flow through intelligent forecasting and predictive analytics. By fostering a holistic approach to AI implementation, industry leaders ensure that the technology‘s impact extends beyond individual departments, driving organization-wide efficiency gains.
Successful AI adopters emphasize accessibility and user-friendly interfaces to maximize ROI. They invest in training programs and intuitive AI tools that empower employees at all levels to leverage AI capabilities effectively. This strategy increases the percentage of staff actively engaging with AI systems, leading to improved productivity and decision-making across the organization.
Top performers in AI adoption consistently measure and iterate on their AI initiatives. They establish clear key performance indicators (KPIs) tied to specific business objectives, tracking metrics such as reduction in operating expenses, improvements in customer satisfaction scores, and increases in revenue per employee. This data-driven approach allows leaders to refine their AI strategies continuously, ensuring optimal returns on their investments.
Extract Lessons Learned From AI Implementation Success Stories
Successful AI implementation stories highlight the importance of integrating predictive analytics throughout business processes. Organizations that effectively leverage AI for forecasting and decision-making often report significant improvements in operational efficiency and revenue growth. By applying scientific methodologies to data analysis, companies can uncover valuable insights that drive
and resource allocation.
Salesforce‘s AI-driven customer relationship managementsystem demonstrates the power of explainable artificial intelligence in enhancing user trust and adoption. By providing transparent explanations for AI-generated recommendations, Salesforce enables users to understand and act upon insights with confidence. This approach has led to increased salesvolume and improved customer satisfaction across various industries.
Companies that prioritize continuous learning and adaptation in their AI implementations tend to achieve sustained
. By establishing feedback loops and regularly reassessing AI models, organizations can refine their algorithms and ensure ongoing relevance. This iterative approach allows businesses to stay ahead of market changes and maintain a competitive edge through data-driven decision-making.
Key Takeaways for Measuring AI ROI Success in Your Organization
Measuring the ROI of AI adoption is crucial for businesses to justify investments and optimize their AI strategies. By setting clear goals, utilizing appropriate calculation techniques, and focusing on essential metrics, organizations can effectively evaluate AI’s impact on efficiency, cost reduction, customer satisfaction, and revenue growth. Beyond financial metrics, considering factors such as employee productivity, market positioning, and long-term strategic benefits provides a comprehensive understanding of AI’s business value. Overcoming challenges in measurement and learning from successful case studies enables companies to refine their
, ensuring sustainable growth and competitive advantage in an increasingly AI-driven business landscape.